https://link-springer-com.ezproxy.ub.gu.se/article/10.1007%2Fs10664-020-09825-8
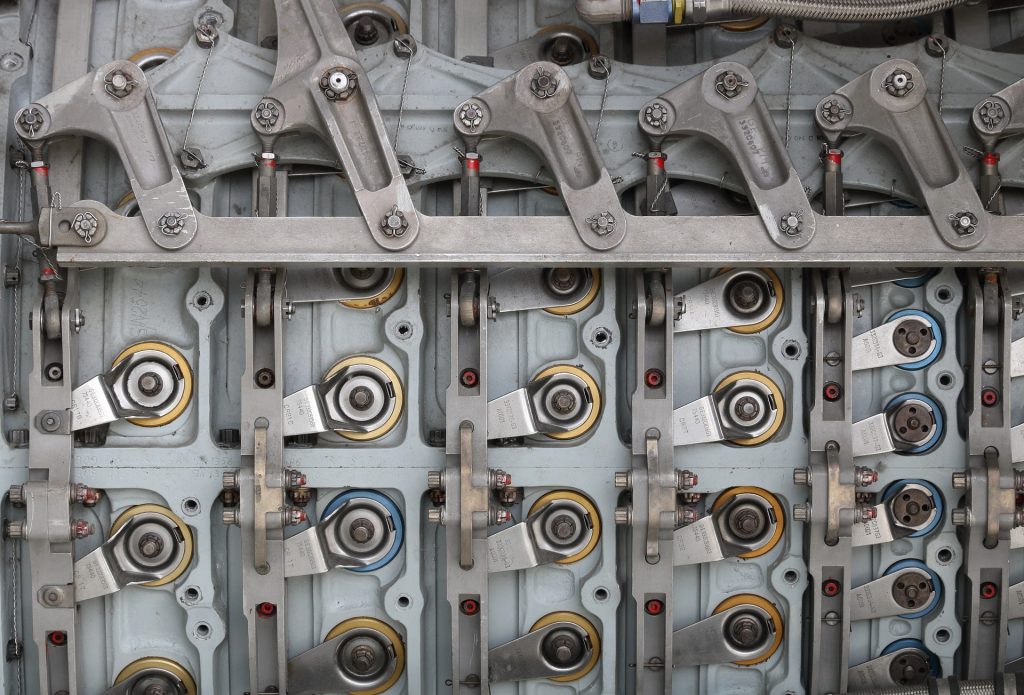
I’ve worked with two great students – Peter and Joshua – who wanted to do something interesting. They developed a tool that could replicate a study from other researchers. However, they did it faster and with less data. We also managed to team up with Mirek from Poznan who improved the classification algorithm and asked his colleagues from new, industrial data.
And this is the outcome – a tool that can connect to a git repository and recognise whether your project is well engineered or not. It helps companies to understand whether their teams are working in a structured manner or ad-hoc.
The tool provides the possibility to assess whether a specific repository is in need for maintenance or not.
Abstract:
Context: Within the field of Mining Software Repositories, there are numerous methods employed to filter datasets in order to avoid analysing low-quality projects. Unfortunately, the existing filtering methods have not kept up with the growth of existing data sources, such as GitHub, and researchers often rely on quick and dirty techniques to curate datasets.
Objective: The objective of this study is to develop a method capable of filtering large quantities of software projects in a resource-efficient way.
Method: This study follows the Design Science Research (DSR) methodology. The proposed method, PHANTOM, extracts five measures from Git logs. Each measure is transformed into a time-series, which is represented as a feature vector for clustering using the k-means algorithm.
Results: Using the ground truth from a previous study, PHANTOM was shown to be able to rediscover the ground truth on the training dataset, and was able to identify “engineered” projects with up to 0.87 Precision and 0.94 Recall on the validation dataset. PHANTOM downloaded and processed the metadata of 1,786,601 GitHub repositories in 21.5 days using a single personal computer, which is over 33% faster than the previous study which used a computer cluster of 200 nodes. The possibility of applying the method outside of the open-source community was investigated by curating 100 repositories owned by two companies.
Conclusions: It is possible to use an unsupervised approach to identify engineered projects. PHANTOM was shown to be competitive compared to the existing supervised approaches while reducing the hardware requirements by two orders of magnitude.