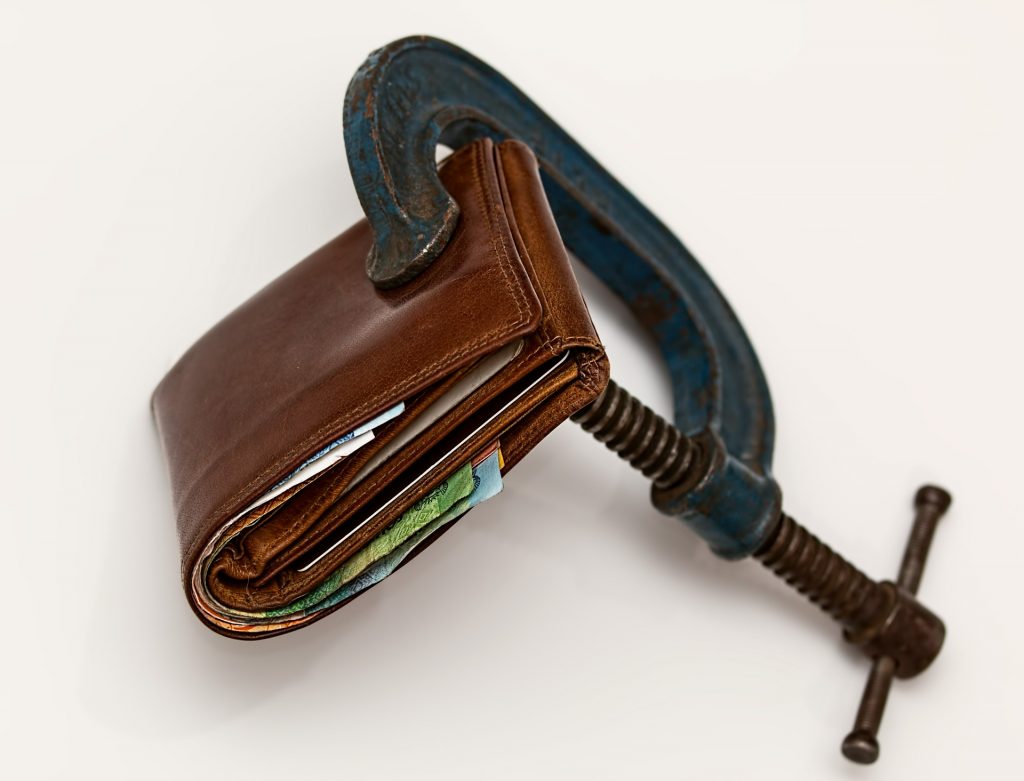
Technical debt is a great metaphor in software engineering. It provides software engineers with the toolkit to communicate how bad design can affect the product in a long run, and how much it can cost to fix these problems. The metaphor has been implemented in many static analysis tools like SonarQube.
Despite its power in communicating, its not clear whether this metaphor is actually useful. It has some dark sides, which makes it a bit tricky to use it. For example, the “conversion” from a problem to the debt, e.g. lack of getter and setter methods to 0.5 days in debt, is one of these challenges. Its also not always clear which of the technical debt categories apply to which products.
What I like about this paper is that it presents a survey of technical debt. For example, it identifies the top causes of technical debt, such as:
- deadlines,
- inappropriate planning,
- lack of knowledge, and
- lack of well-defined process.
These challenges are present in most companies today, and the first two – deadlines and inappropriate planning – are often associated with start-ups and agile organizations. I recommend to take a closer look at the mindmap in the paper (Fig. 5) to dive deeper into the causes.
Quote from the abstract: We identified a total of 78 causes and 66 effects, which confirm and also extend the current knowledge on causes and effects of TD. Then, we organized the identified set of causes and effects in probabilistic cause-effect diagrams. The proposed diagrams highlight the causes that can most contribute to the occurrence of TD as well as the most common effects that occur as a result of debt.