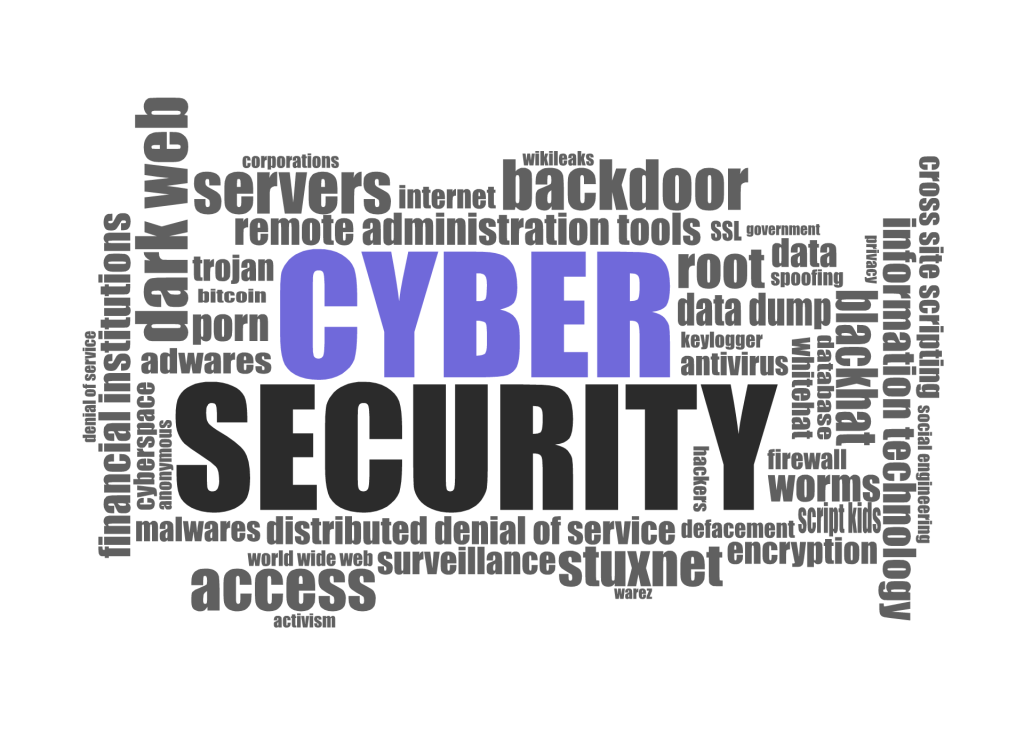
sec23summer_449-mirsky-prepub.pdf (usenix.org)
Cybersecurity has been, and will always be, a challenge for software systems. It is also perceived as an art when it comes to security analysis (or exploitation for that matter). There is no single tool, no single method that will make our software secure.
This article is interesting because of the way that it works. Usually, security analyzers are token-based analyzers which see programs as a set of instructions. They are very good, but they struggle with understanding the context of the analyzed program.
Let me give you an example. We’re analyzing a program for SQL injections – a very simple vulnerability. We can check that the SQL statement in the code contains any parameters. If it does not, then it’s safe – we know what we do with the database, but it’s not very common (or even useful). So, most statements will have some sort of parameters, and this is where the tricky part is. These parameters need to be validated, but this validation can be done in the same function (just before the actual SQL statement) or it can be done somewhere in the calling function/method. The check in the calling function/method is the part where token-based security analyzers give up.
Now, this paper presents an approach which works on a call graph, which allows for this interesting checks. I still need to understand it myself, but I hope to do it quite soon. The full source code is available here: GitHub – ymirsky/VulChecker: A deep learning model for localizing bugs in C/C++ source code (USENIX’23)