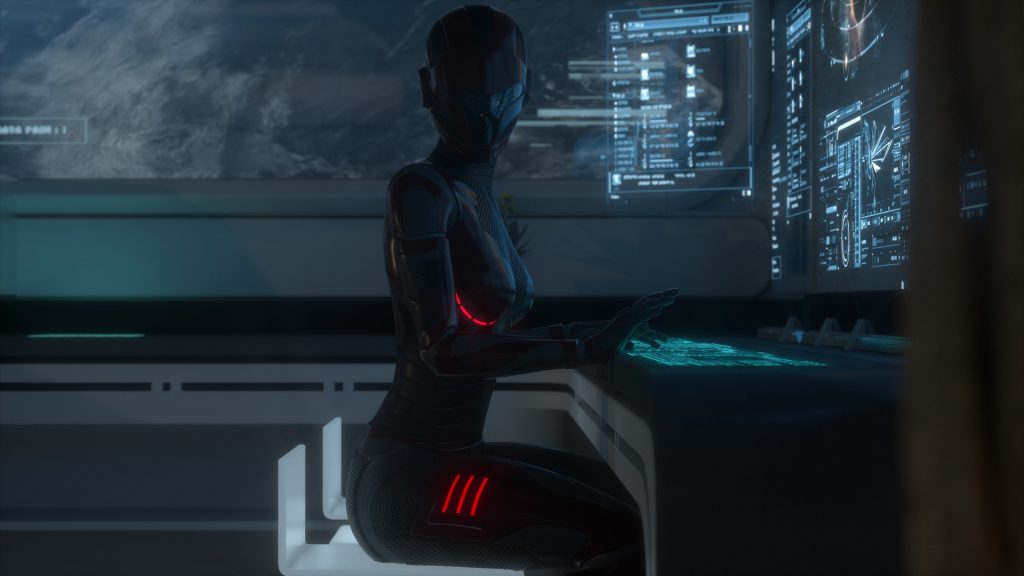
Sparse reward for reinforcement learning‐based continuous integration testing – Yang – – Journal of Software: Evolution and Process – Wiley Online Library
This is the last post that I want to write in 2021. The year has been hectic and full of surprises. First, we got the news that the vaccine works for Covid-19. We all prepared for normalization, for being able to travel, visit friends, families, and conferences in person.
Then came the new variants, like the Omikron, which seem to escape from the vaccine, and countries still are not ready for opening. Conferences get postponed, trips canceled. I hope this is just a temporary situation and that we will be able to get in control of the situation again.
For the last post in 2021, I chose one of the articles that I’ve recently read – about the use of reinforcement learning in integration testing. Kind of a different approach to what we do in the Software Center project.
This paper tackles the problem of sparse rewards for fitness functions when using reinforcement learning for test selection. It proposes a combination of historical data and a function that assigns a higher reward for non-sparse data. It looks like the work is very promising, as it has been tested on 14 different industrial data sets. I need to check if during the coming holidays. It’s a project to do for X-Mas
With that, I would like to thank all of you for being here with me during 2021 and hope that we can continue in 2022. Wish you all great holidays and the best of luck in the coming 2022!
From the abstract:
“Reinforcement learning (RL) has been used to optimize the continuous integration (CI) testing, where the reward plays a key role in directing the adjustment of the test case prioritization (TCP) strategy. In CI testing, the frequency of integration is usually very high, while the failure rate of test cases is low. Consequently, RL will get scarce rewards in CI testing, which may lead to low learning efficiency of RL and even difficulty in convergence. This paper introduces three rewards to tackle the issue of sparse rewards of RL in CI testing. First, the historical failure density-based reward (HFD) is defined, which objectively represents the sparse reward problem. Second, the average failure position-based reward (AFP) is proposed to increase the reward value and reduce the impact of sparse rewards. Furthermore, a technique based on additional reward is proposed, which extracts the test occurrence frequency of passed test cases for additional rewards. Empirical studies are conducted on 14 real industry data sets. The experiment results are promising, especially the reward with additional reward can improve NAPFD (Normalized Average Percentage of Faults Detected) by up to 21.97%, enhance Recall with a maximum of 21.87%, and increase TTF (Test to Fail) by an average of 9.99 positions. “