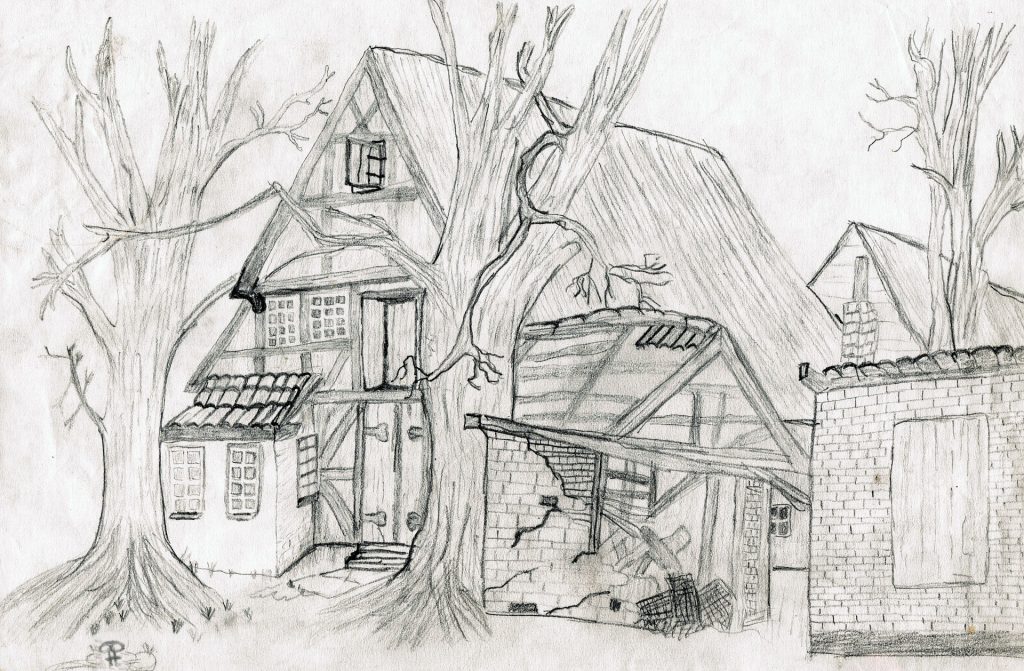
https://www.computer.org/csdl/proceedings-article/models/2023/248000a173/1SOLExN0XaU
It’s been a while since I worked with models and I looked a bit at how things have evolved. As I remember, one of the major problems with modelling was one of its broken promises – simplicity.
The whole idea with modelling was to be able to sketch things, discuss candidate solutions and then to transfer them on paper. However, in practice, this never worked like that – the sheer process to transfer a solution from the whiteboard to a computer took time. Maybe even so much time that it was not really worth the effort of informal sketches.
Now, we have CNNs and all kinds of ML algorithms, so why not use that? This paper studies exactly this.
The paper “SkeMo: Sketch Modeling for Real-Time Model Component Generation” by Alisha Sharma Chapai and Eric J. Rapos, presents an approach for automated and real-time model component generation from sketches. The approach is based on a convolutional neural network which can classify the sketches into model components, which is integrated into a web-based model editor, supporting a touch interface. The tool SkeMo has been validated by both calculating the accuracy of the classifier (the convolutional neural network) and through a user study with human participants. At the moment, the tool supports classes and their properties (including methods and attributes) and relationships between them. The prototype also allows updating models via non-sketch interactions with models. During the evaluation the classifier performed with an average precision of over 97%. The user study indicated the average accuracy of 94%, with the maximum accuracy for six subjects of 100%. This study shows how we can successfully employ machine learning into the process of modeling to make it more natural and agile for the users.