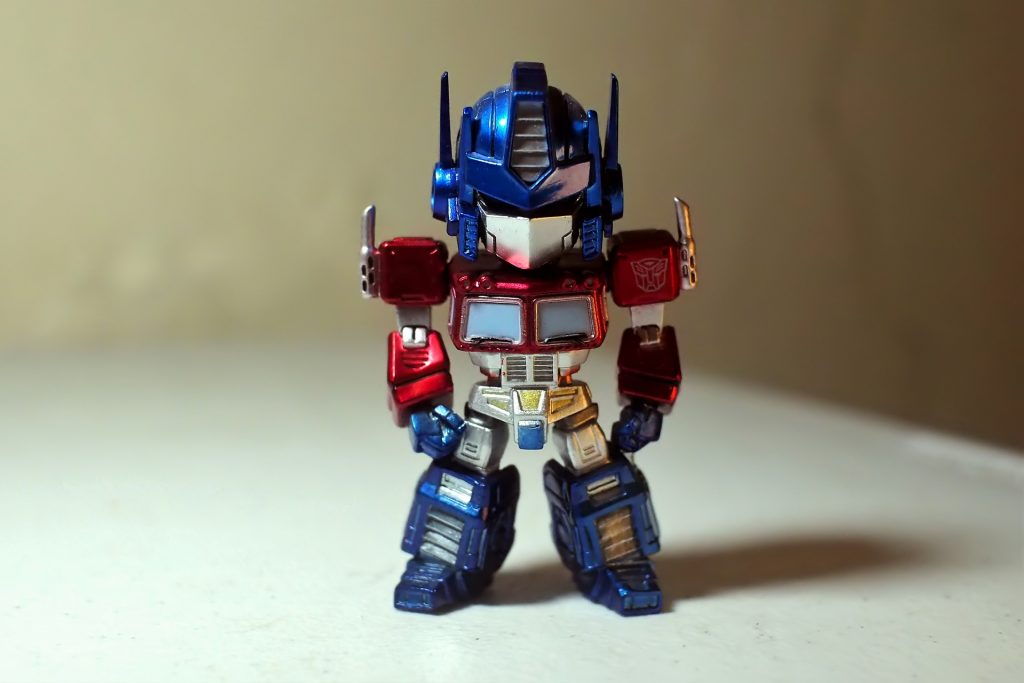
AutoML, a promise of green pastures, less work, optimal results. So, it is like that? In this post I share my view on this and experience from running the first test using that model.
First of all, let’s be honest, there is not such thing as a free lunch. In case of autoML (auto-sklearn), the price tag comes first with the effort, skills and time to install it and make it work. The second is the performance…. It’s painfully slow compared to your own models, simply because it tests a lot of models here and there. It also take a lot of time to download and to make it work.
But, first thing first, let me tell you where I start. So, I used the data from the MicroHRV project ( 3. MicroHRV: Recognizing Rare Events in Microwave Radio Links and Intensive Care Units using Machine Learning – Software Center (software-center.se)). The data is from patients being operated to remove clots of blood from the brain (although dangerous it may sound, the actual procedure is planned and calm). I wanted to check whether autoML can do better compared to what we have at the moment.
What we have at the moment (for that particular dataset) is: Accuracy: 0.98, Precision: 0.98, Recall: 0.98 – using Random Forest classifier. So, this is actually already very good. For the medical domain, that’s actually in class of its own, given our previous studies ended up with ca. 0.7 in accuracy at best.
When it comes to installing autoML – if you like stackoverflow, downgrading, upgrading, compiling, etc. and run Windows 10, then it’s your heaven. If you run Linux – no problems. Otherwise – stick to manual analyses:)
After two days (and nights) of trying, the best configuration was:
- WSL – Windows Subsystem for Linux
- Ubuntu 20, and
- countless of oss libraries
It takes a while to get it to work, the question is whether the results are good enough…
After three hours of waiting, a lot of heat from my laptop, over 1,000 models tested resulted in Accuracy: 0.91, Precision: 0.94, Recall: 0.91
So, worse than my manual selection of models. I include the confusion matrices.
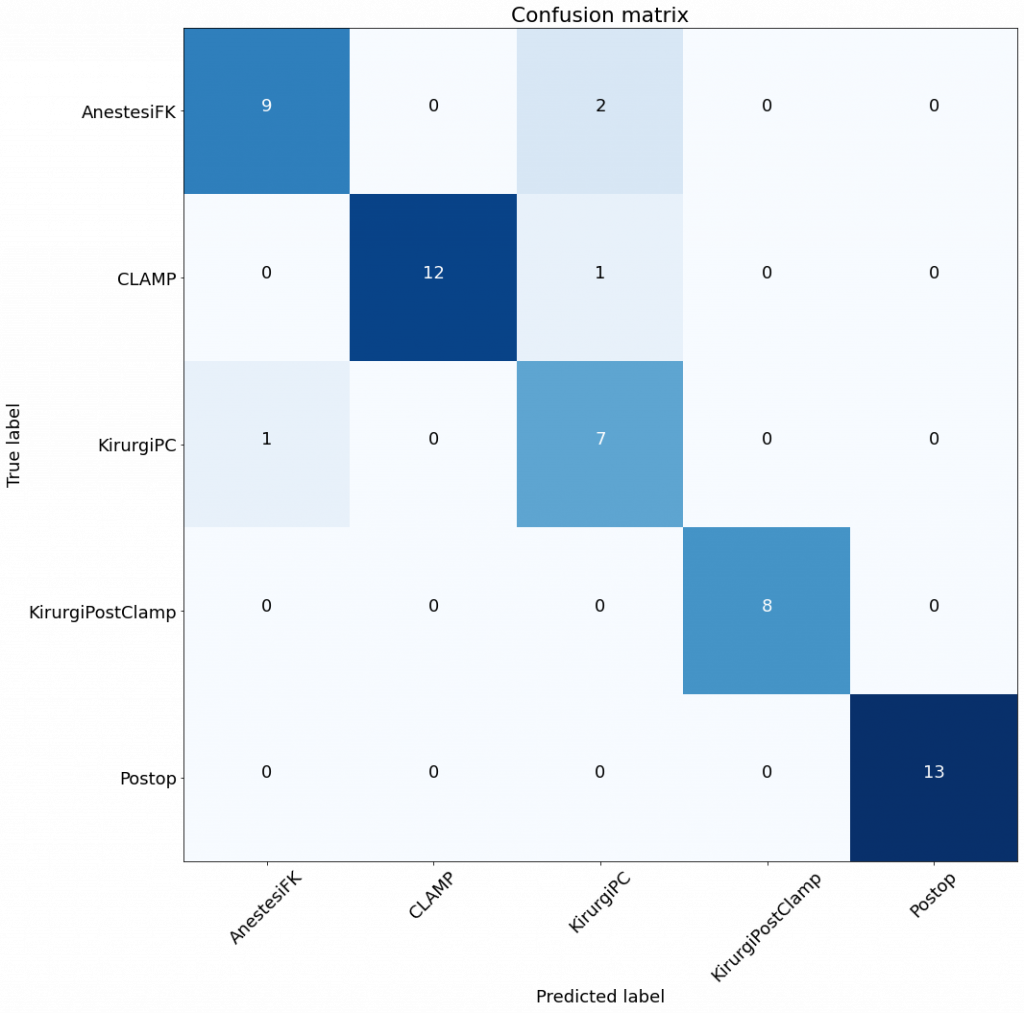
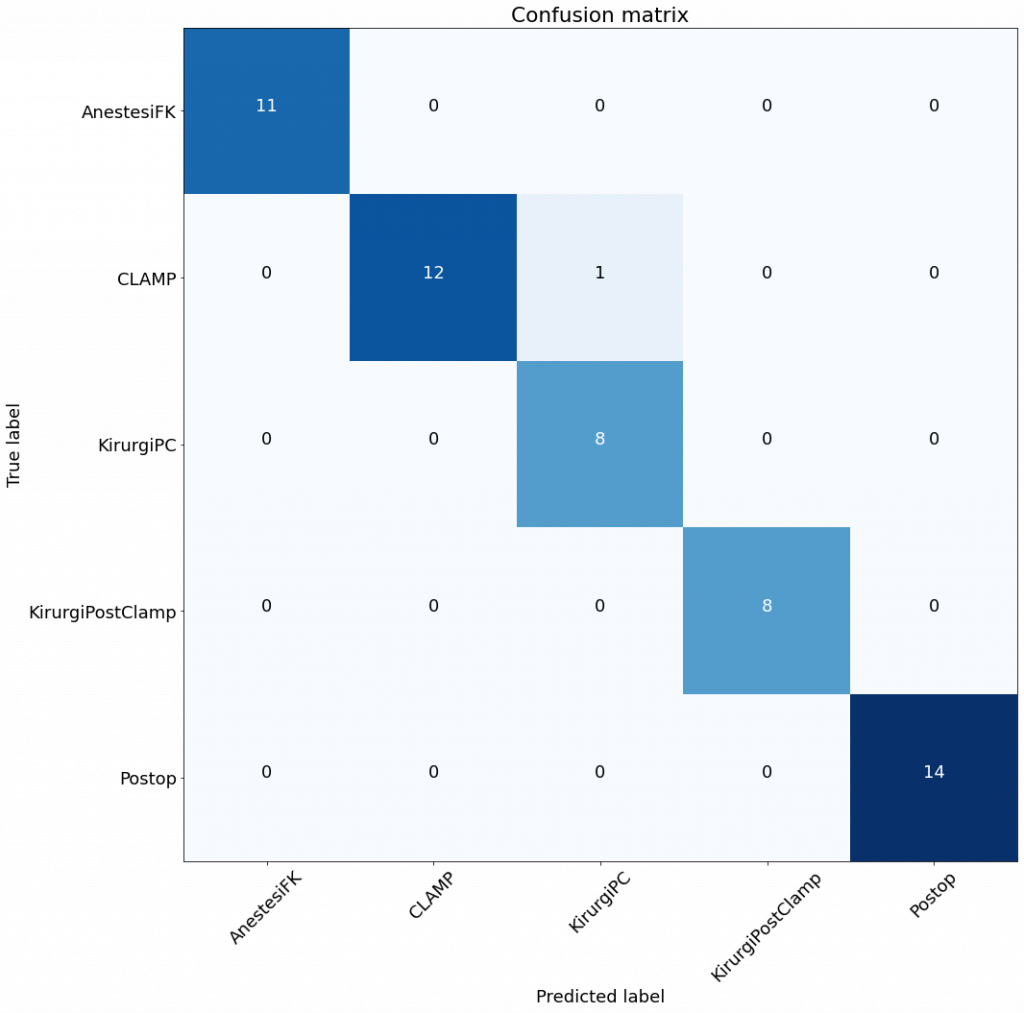
The matrices are not that different, as the validation sets are not that large either. However, it seems that the RF is still better than the best model from autoML.
I need work more on that and see if I do something wrong. However, I take this as a success – I’m better than autoML (still some use of an old professor) – instead of a let-down of not getting better results.
By the end of the day, 0.98 in accuracy is still very good!