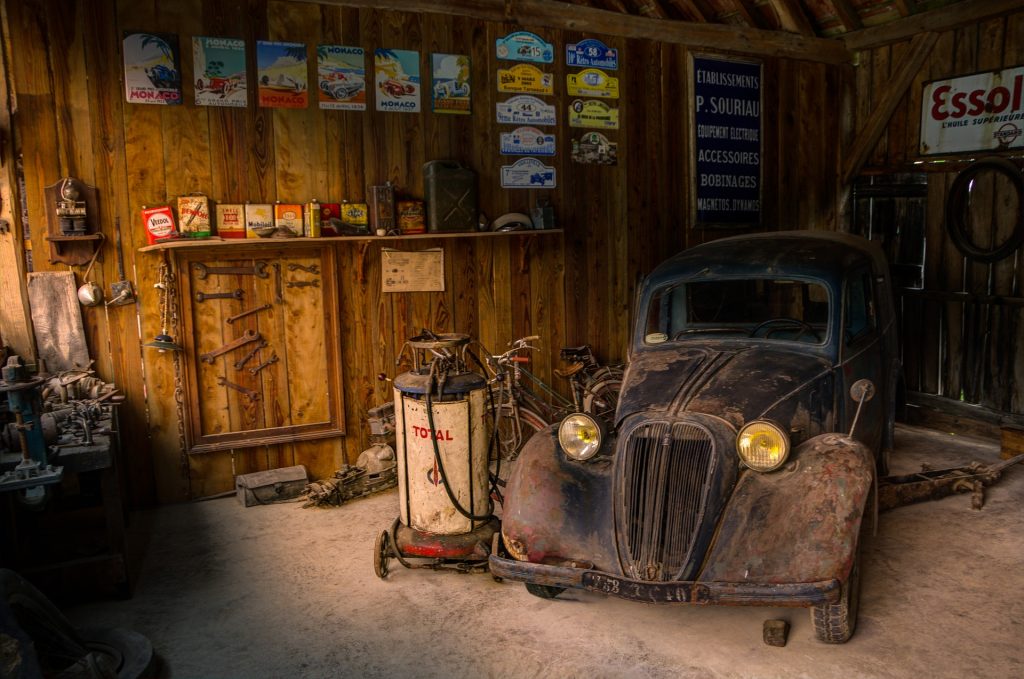
ICSE2021_B.pdf (igor.pro.br)
For many of us, software engineering is the possibility to create new projects, new products and cool services. We do that often, but we equally often forget about the maintenance. Well, maybe not forget, but we deliverately do not want to remember about it. It’s natural, as maintaining old code is not really anything interesting.
When reading this paper, I’ve realized that my view about the maintenance is a bit old. In my time in industry, maintainance was “bug-fixing” mostly. Today, this is more about community work. As the abstract of this paper says: “Although Open Source Software (OSS) maintainers devote a significant proportion of their work to coding tasks, great maintainers must excel in many other activities beyond coding. Maintainers should care about fostering a community, helping new members to find their place, while also saying “no” to patches that although are well-coded and well-tested, do not contribute to the goal of the project.”
This paper conducts a series of interviews with software maintainers. In short, their results are that great software maintainers are:
- Available (response time),
- Disciplined (follows the process),
- Has a global view of what to achieve with the review,
- Communicative,
- Emapthetic,
- Community building,
- Technically excellent,
- Quality aware,
- Has domain experience,
- Motivated,
- Open minded,
- Patient,
- Diligent, and
- Responsible
It’s a long list and the priority of each of these characteristics differs from one reviewer to another. However, it’s important that we see software maintainer as a social person who can contribute to the community rather than just sit in the dark office and reads code all day long. The maintainers are really the persons who make the software engineering groups work well.
After reading the paper, I’m more motivated to maintain the community of my students!