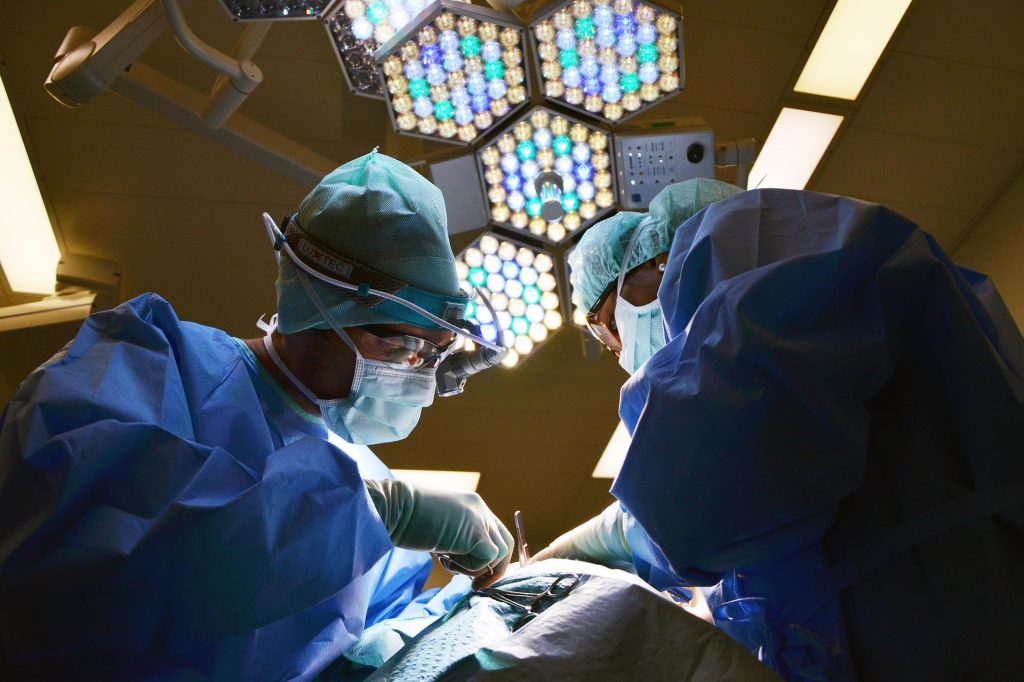
Identifying and classifying anomalies in software engineering data is a well-known field. Using ML to identify intrusion attacks, credit card frauds, defects in production systems – are just a few of the examples of how broad the field is. Wherever we have data, we can have anomalies.
Both types of anomalies have similarities, but also differences, which provides us with an opportunity to study which of the algorithms for anomaly detection work best. We tried both the ML algorithms and domain-specific ones. Well, spoiler alert – not much has actually worked.
In our project together with Sahlgrenska University Hospital and Chalmers AI Research Center ( Chalmers AI Research Centre – Chair | Chalmers ), anomalies come in two shapes. One type of anomaly is the set of disturbances in radio networks, such as rain or wind. The other type is a specific type of event during surgeries, such as clamping of the carotid artery.
What works, on the other hand, is when we pivot on the problem. Instead of identifying anomalies, we can search for anomalies of a specific type. Instead of defining an anomaly as something deviating from the normal operations, we can say that we look for specific, though rare, events.
So far, we can identify anomalies pretty well and we work on being better to classify them automatically. So stay tuned if you would like to know more.