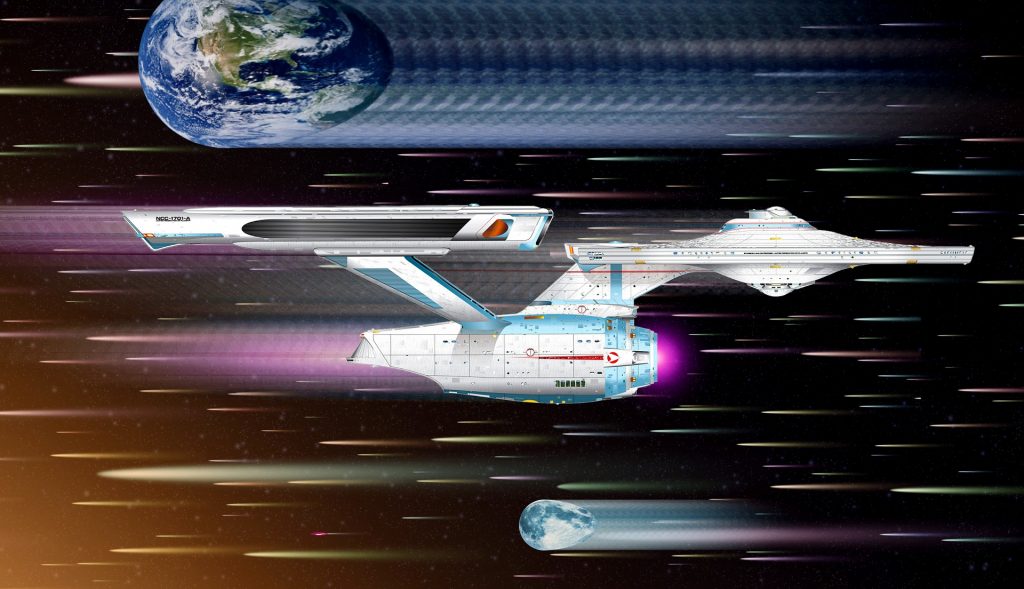
While on vacation, I managed to watch a number of sci-fi movies, which I wanted to watch but did not have the time during the academic year. This got me thinking about certain laws of physics and the laws of software engineering. I think there are many similarities, and let me start by considering the speed of light, as a starter.
First of all, what we know about the speed of light is that it’s the fastest we know and that Einstein’s theory of relativity says that we cannot travel faster than the speed of light. Even if we were, such a speed is tremendously difficult.
- How would we know where we travel if we cannot see where we travel (we go as fast as we can see, literally). So, the travel would be very fast and, probably, very short.
- If we, somehow, see where we go, or detect an obstacle (e.g. by knowing its predicted position based on prior observations), how can we steer? We’re going so fast, that for the majority of the travel time, we would be going in straight lines. These straight lines would be similar to either the hyperjumps from Star Wars or the clicks from the Guardians of the Galaxy.
- If we’re literally the fastest objects, how can others see us and avoid is? Is it possible to avoid the light? No, it’s not possible. This means that we would be super-chaotic.
Therefore, I think that, even if we could, traveling at the speed of light is probably not the best idea. At least not conceptually, which may change as we change.
How does it affect the laws of software engineering then. Well, I would start with the laws of complexity.
For a while now, I’ve been broadcasting the opinion that the complexity of software cannot be reduced, it can only be hidden. For complex problems, we need complex software and complex software cannot be simple. If our algorithms have many conditions, we cannot take them away, we can hide them in functions, but never get rid of them. That’s the first parallel – we cannot travel faster than the speed of light.
We can hide complexity, and thus make the program/software easier to understand and maintain, but the better we hide it, the harder it is to avoid/predict complexity. Packaging complex algorithms in simple blocks will make it difficult to make modifications. Actually, not to make the modifications, but to overview the consequences of these modifications.
If we simplify the program/algorithm too much, we need to expect that it’s going to provide erroneous results for some cases – again, complex problems require complex programs. An example of such issue is approximating continuous functions – since our computers are discrete, there is always some degree of error in such an approximation.
Finally, interconnectivity and modularity as a means of handling complexity have their limits. I do not think we can develop increasingly complex software by increasing its size. I believe it’s going to be difficult in the long run. We need to make sure that we have the competence to handle complexity and we need to be able to make the complexity apparent.