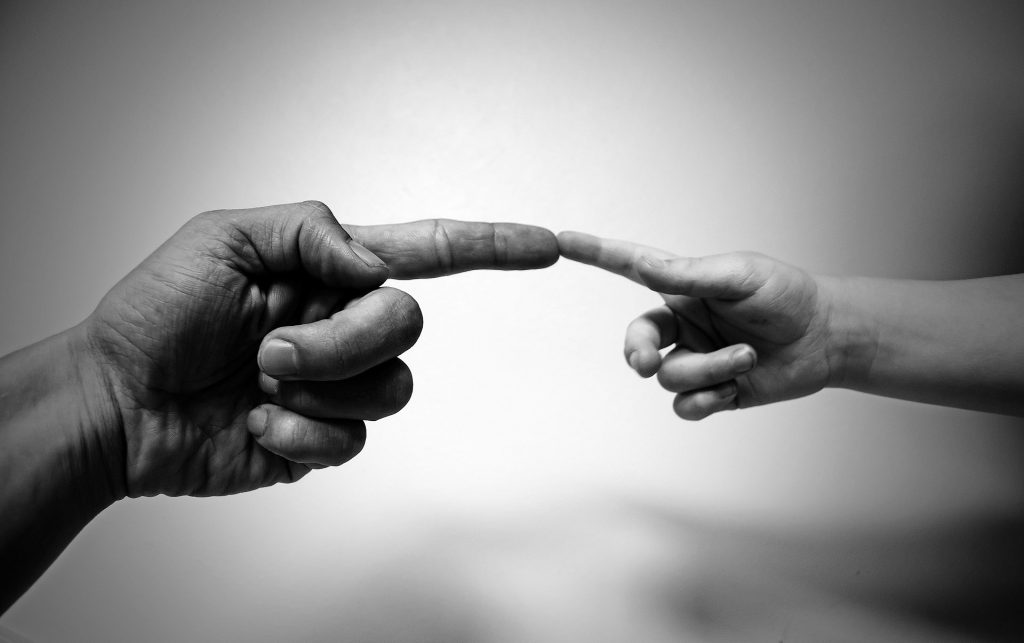
Image by PublicDomainPictures from Pixabay
https://dl.acm.org/doi/pdf/10.1145/3664805
In artificial intelligence (AI), the conversation is shifting from mere technological advancements to the implications these innovations have on society. The paper “Human-Centric Artificial Intelligence: From Principles to Practice” focuses on the concept of designing AI systems that prioritize human values and societal well-being. It’s not my usual reading, but it caught my attention because of the title close to one of the programs that our faculty has.
Key Principles of Human-Centric AI
The paper outlines several core principles necessary for the development of human-centric AI:
- Transparency: AI systems must be transparent, providing clear insights into how decisions are made.
- Fairness: Ensuring that AI systems operate without bias and are equitable in their decision-making processes.
- Accountability: Developers and organizations must be accountable for the AI systems they create. This involves implementing mechanisms to monitor AI behavior and mitigate harm.
- Privacy: Protecting user data is paramount. AI systems should be designed to safeguard personal information and respect user privacy.
- Robustness: AI systems must be reliable and secure, capable of performing consistently under varying conditions and resilient to potential attacks.
It seems to me that the journey towards human-centric AI is still not taken, we have not achieved our goals. Balancing innovation with ethical considerations can be difficult, especially in a fast-paced technological landscape.
As we continue to integrate AI into more products, services and thus various aspects of society, the emphasis on human-centric principles will be crucial in ensuring that these technologies benefit humanity as a whole. We need to keep an eye on these developments.