In 2015 I had a chance to co-organize a workshop on automotive software architectures. The workshop brought together many researchers and practitioners to discuss the issues related to designing large automotive software systems. After the workshop I realized that there is still a need to describe how to design the automotive software from the perspective of a software engineer.
Thanks to my editor, Ralf, who approached me and asked for a book on automotive software architectures, we have a possibility to show the results of it.
The book is primarily for software engineers who want to understand how the automotive software is designed, deployed and standardized.
Abstract:
This book introduces the concept of software architecture as one of the cornerstones of software in modern cars. Following a historical overview of the evolution of software in modern cars and a discussion of the main challenges driving that evolution, Chapter 2 describes the main architectural styles of automotive software and their use in cars’ software. In Chapter 3, readers will find a description of the software development processes used to develop software on the car manufacturers’ side. Chapter 4 then introduces AUTOSAR – an important standard in automotive software. Chapter 5 goes beyond simple architecture and describes the detailed design process for automotive software using Simulink, helping readers to understand how detailed design links to high-level design. Next, Chapter 6 presents a method for assessing the quality of the architecture – ATAM (Architecture Trade-off Analysis Method) – and provides a sample assessment, while Chapter 7 presents an alternative way of assessing the architecture, namely by using quantitative measures and indicators. Subsequently Chapter 8 dives deeper into one of the specific properties discussed in Chapter 6 – safety – and details an important standard in that area, the ISO/IEC 26262 norm. Lastly, Chapter 9 presents a set of future trends that are currently emerging and have the potential to shape automotive software engineering in the coming years.
This book explores the concept of software architecture for modern cars and is intended for both beginning and advanced software designers. It mainly aims at two different groups of audience – professionals working with automotive software who need to understand concepts related to automotive architectures, and students of software engineering or related fields who need to understand the specifics of automotive software to be able to construct cars or their components. Accordingly, the book also contains a wealth of real-world examples illustrating the concepts discussed and requires no prior background in the automotive domain.
Available at Amazon: https://www.amazon.com/dp/3319586092/sr=8-1/qid=1502048739/ref=olp_product_details?_encoding=UTF8&me=&qid=1502048739&sr=8-1
And Springer: http://www.springer.com/la/book/9783319586090
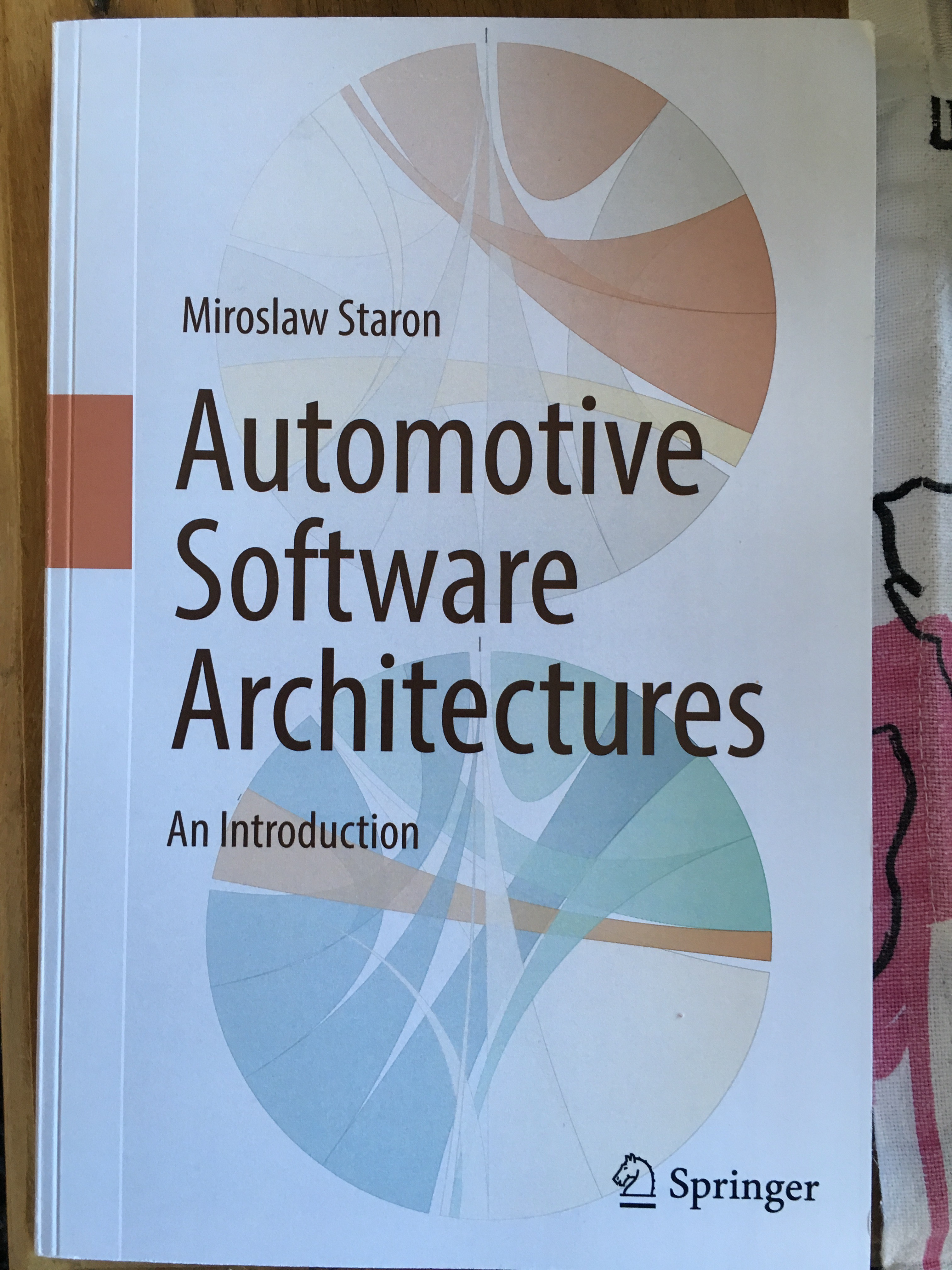